Coaching & Analytics: Lineup Data (Newsletter #3)
From the archives: V1 of the old Hoop Vision coaching newsletter
NOTE: This post was originally sent as an email to subscribers in July 2017; the Hoop Vision newsletter and operation was far different at that time. Regardless, enjoy…
The Rise of Lineup Data
In March 2013, Pete Thamel wrote a profile about then Butler graduate assistant Drew Cannon and his use of statistics alongside Brad Stevens. The main analysis explained in the article: Lineup Data.
Stevens and Cannon, according to the article, used data to decide substitution patterns and "rules". The article also talked about the emergence of analytical thinking in the NBA, with Stevens predicted a similar emergence in the NCAA. He said, "Whenever you publish this article, it's going to change".
Four years later, Stevens certainly wasn't wrong. That article had a tremendous impact on the NCAA. However, for a lot of programs the need for data analytics wasn't exactly the takeaway from the article. Instead, the key takeaway was the need for lineup data.
If your email is listed on a NCAA men's coaching staff website, you are likely getting emails on a weekly basis from different consultants and companies offering lineup data. And these sales pitches are for good reason:
Coaches love lineup data. In fact, coaches are making decisions based off lineup data.
What is it?
The first iteration of lineup data was simply plus-minus, which is the team's point differential when a given player is on the floor. More current lineup data no longer just looks at one player. It also looks at 2-man, 3-man, 4-man, and 5-man lineups. Current lineup data also goes beyond just point differential. Theoretically, it can answer questions like "How does my offensive rebounding change when Player X and Player Y are in the front-court together?"
The magic of lineup data, and why coaches love it so much, is that it is measuring team impact. Players bringing hustle and "intangibles" to a team that don't show up in the box score should be properly credited by lineup data. For a coach, it's a very objective way to explain playing time (or lack of playing time) to a player.
For the reasons above, analytics in college basketball has essentially turned into lineup data. Coaches can be very skeptical of new stats and often use context to rip it apart, but for whatever reason lineup data has avoided this usual scrutiny.
Misuse in Decision Making
In reality, lineup data can be highly contextual. Because the games are only 40 minutes and you are only playing two games a week, NCAA bench players are often times only used by necessity. This obviously contrasts the NBA, where teams tend to have a set rotation that can be used to extract meaningful data for bench players. In the NCAA, a backup point guard may only play meaningful minutes if the starter is hurt or in foul trouble.
Even for teams that use their bench in a more robust manner, sample size is extremely small in the NCAA. Advanced NBA models — which account for contextual factors like opponent strength — often use multiple years of data in order to make predictions based off of lineup data. That type of sample size doesn't exist at the college level.
To illustrate the limits of lineup data, I used HoopLens.com to compare data from the first half of Big East play to the second half. For this (admittedly crude) study, I went player-by-player in the Big East to see how his team performed offensively with him on the court in the first nine games of conference play. I accounted for team strength by comparing the player's performance to the team's average performance. I then did the same exact thing for the second nine games of conference play.
The question I'm looking to answer here is, "If a team was especially efficient with a certain player on the court during the first half of conference play, did that trend continue in the second half?" The answer is no:
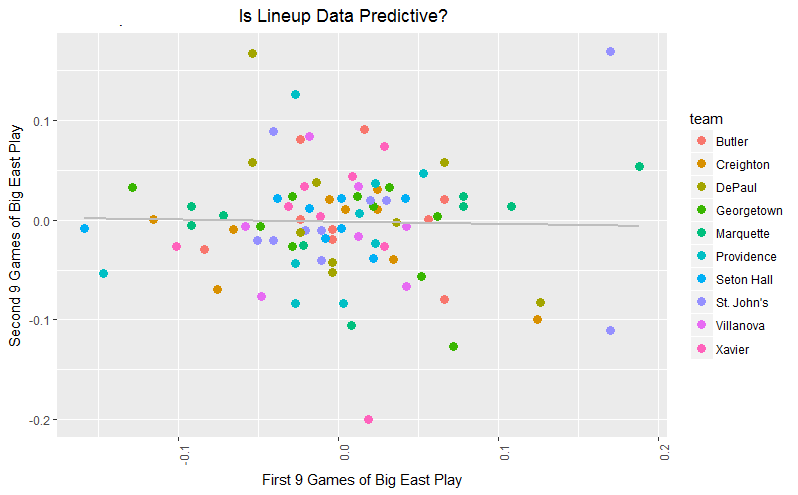
The trend line is basically flat, meaning correlation between the first 9 game and last 9 games is non-existent. The study is by no means rigorous enough to throw out lineup data altogether. However, in small samples a team's average points per possession is consistently a stronger predictor of future player performance than that player's points per possession.
Using Lineup Data Effectively
Although it is of limited utility as a predictor, lineup data can be quite useful when used responsibly.
Let's say a player or unit has poor offensive numbers according to lineup data. The reaction by a coach should not simply be to stop playing that player/unit. Instead, it should be to ask why. Instead of using it as a predictor, lineup data is best used as an organizer.
With the rise of the three-point line, everyone wants to go small for floor spacing. This can lead to players playing positions without getting practice reps at that position. A guard asked to play the 4 probably doesn't have a great feel for running plays at that position, and chances are the plays aren't maximizing his abilities anyways.
The data just describes the past effectiveness of the lineup. The coaching staff can utilize that data (and film) to determine both the why and how to improve.
I think a great way to think of lineup data is like a Synergy statistic. Synergy classifies all of your possessions and can tell you performance on (for example) spot-up shot attempts. It then allows you to click on that number and watch all of your spot ups. So while it's helpful to know that you are below average in a category, the real value is looking at the numbers and film to do some critical thinking on why and how to improve.

If your efficiency on spot up attempts is low, you wouldn't simply ban spot ups. You would evaluate the plays and players contributing to those attempts and tweak strategy as necessary. Lineup data is no different. With any given lineup there are many factors and decisions to consider:
How do you defend ball screens? What offense do you run to maximize the five on the court? Do you crash the glass or set up your defense? How fast are you trying to play?
Instead of using lineup data (and film) to make a binary yes/no decision on a particular unit or player, the best staffs use it to evaluate and improve.