Hoop Vision Weekly: Individual Defense (7/28/19)
The struggles and future of evaluating an individual player's defensive value
Mailbag Q&A coming next week
Big thanks to everyone for spreading the word about the newsletter last week! It’s much appreciated as we continue to grow.
As previously mentioned, next week’s edition will be a Q&A. Please send your questions on just about anything basketball related. The more esoteric the better—and we’d expect nothing less from this community of people diving deep into college basketball scheme and analytics in late July.
In this edition:
A summary of Nate Silver’s new defensive metric DRAYMOND
Defensive statistical modeling and its place in coach decision making
The “numerical eye-test” and a defensive accounting report we used at NMSU
The future of defensive analytics
New metric, same skepticism
Earlier this month, 538 and Nate Silver presented a new NBA defensive metric called DRAYMOND.
“[DRAYMOND] does get at one essential discovery we made in playing around with the opponents’ shooting data: the idea of minimizing openness. The main goal of shooting defense, especially in today’s spacing-centric, ball-movement-forward offensive era, is really to minimize the chance of an open shot.”
The reaction to DRAYMOND—at least on my Twitter timeline—was one of nearly unanimous discontent, but all seemingly for different reasons.
Some of the different criticisms included:
It’s already been done before by others in the basketball analytics space
The data being used to build the metric is “highly imperfect”
It has some questionable “metric design choices”
It’s just a “feature” to be used in a model, not a catch-all defensive statistic
The assumptions made about defense the metric are built on are flawed
Quantifying individual defense is extremely hard. The purpose of this newsletter isn’t necessarily to get into detail about DRAYMOND. This is, of course, a college basketball newsletter - and the data set used for DRAYMOND isn’t available at the college level.
Instead, we’ll take take a look at the overall state of evaluating individual defense—both from an analytics perspective and coaching perspective.
Often times the tension between analytics and film are overstated. But when it comes to individual defense, I actually think there are some real issues causing conflict that are worth discussing.
Let’s start by taking a look at both sides, followed by some thoughts on how to alleviate the tension.
The disconnect between statistical models and coaching
If you head over to kenpom.com — or any other college basketball stats website — you won’t find a lot of information about an individual player’s defensive skill set.
Block percentage is probably your best bet at determining some type of defensive role. It’s somewhat safe to say that players with high block percentages are “rim protectors,” but even that still leaves a large leftover group of “non-rim protectors” we don’t know much about.
This isn’t an indictment on analytics or the people performing the analysis. Instead, it’s just an issue that is inherent to defense. The process of trying to prevent a team from scoring (defense) doesn’t produce as many relevant box score statistics as the process of trying to score (offense).
With that in mind, statistical modelers have used different ways to measure defensive impact. The first way is the idea of a proxy variable, defined by Wikipedia as:
A variable that is not in itself directly relevant, but that serves in place of an unobservable or immeasurable variable.
The proxy is, in my mind, one of the root causes of disagreement in basketball analysis.
The best variable to illustrate this is steals. In a vacuum, a steal is an intrinsically positive result for the defense.
But there’s also a risk to gambling for steals - and a coach’s defensive scheme helps determine a team’s overall risk tolerance. Over the last five years of Bo Ryan’s career, for example, his best team finished 279th in forcing turnovers - and that was by design.
It would be quite easy to find a coach with strong opinions on why steals are not necessarily indicative of a good defensive player. Still, they tend to increase the predictive power of statistical models measuring player value.
The concept of the proxy variable is a big reason why. Regardless of the value of a steal in and of itself, it can be a proxy for other variables. Players accumulating steals might be more likely to have superior physical traits like: agility, athleticism, and wingspan.
Those physical traits are going to help in areas that a coach would unanimously agree are representative of good defense: keeping the ball in front of you, strong close-outs after helping, and being slippery on screens.
It’s not that we can’t measure those three variables (more on that later). It’s that the box score doesn’t. So we turn to proxies instead.
That’s not to say statistical models are bad. In fact, the ability to use proxies to algorithmically extract information and make predictions can just as easily be seen as an advantage of modeling.
The model also has the advantage of being free from pre-conceived notions and coaching axioms - like about steals. It’s making objective evaluations based on the data.
The problem here is more specific to what’s useful for a coach. Defensive models are quantifying “value,” but with very little information on why. The use of proxies only makes the why more confounding.
If your statistical model produces a counter-intuitive result and you’re going to recommend that counter-intuitive suggestion to a coach, you’d better have a good reason why.
Defensive accounting and “the numerical eye-test"
At New Mexico State, we charted our own defensive statistics after each game. I personally called it the “defensive accounting” report, but it had a similar function to the box score.
The defensive accounting report was really just a numerical extension of the eye-test. We would watch every possession from the previous game and assign every point allowed and turnover forced to:
The player (or in some cases multiple players - points could be divided) responsible
The specific action (ball screen, 1 on 1, help) responsible
The defensive accounting report from one of our biggest wins of the season, against then #6 in the country Miami, is below.
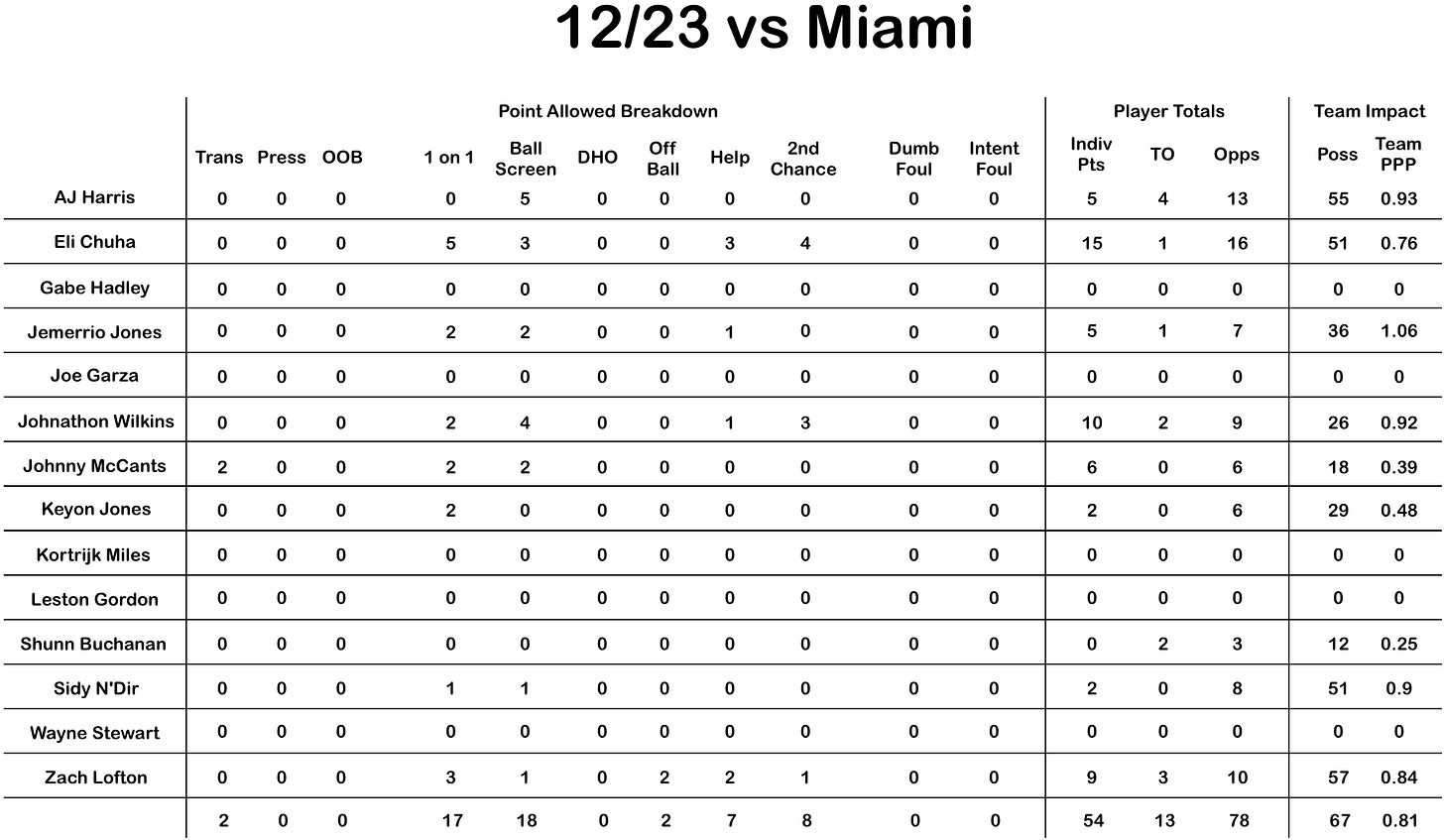
The bottom row shows that we gave up 17 points off 1-on-1’s, 18 points off ball screens, and so on.
The “Indiv Pts” column shows which players were responsible for the most points.
The “Opps” column is opportunities - essentially a tally of how many times that player would have been held responsible for points (even if the shot didn’t go in).
The biggest advantage to the defensive accounting report is that it was done by someone with knowledge of our scheme and gameplan. We knew what was supposed to happen on a given play, so we were better equipped to assign credit/blame.
The report is really nothing more than a numerical eye-test. Instead of watching the film and just simply saying “Eli can’t let the roller get behind him there,” you’re just going a step further and assigning the two points to Eli.
Just like with statistical modeling, there are pros and cons to this type of method. Unsurprisingly, we used the defensive accounting report to hold our players accountable. It was a good descriptive look at who messed up the most on the defensive side of the floor.
For a coach, the exercise of creating something like a defensive accounting report should cause a bit of doubt around the eye-test. If not, that coach is likely vastly overestimating his/her ability to process the game.
As coaches, we tend to focus on the execution of our five players on the floor in a very narrow sense. And that’s probably for good reason: the execution is what you can control. But there are so many different variables that determine the result of a possession - and they’re all in a constant state of change as the possession progresses.
The type of information processing ability needed to watch and evaluate defense is, somewhat ironically, a task truly fit for a computer; as the basketball analytics revolution continues, machine learning and artificial intelligence will likely take center stage on the defensive end.
The future of relevant defensive analytics for coaches
In some ways, I think the defenders of the “eye-test” are right. A well-trained basketball mind can likely evaluate a player’s defensive value better than the current public basketball metrics.
The mistake being made by the eye-test defenders is believing that the machines won’t catch up.
Second Spectrum already has computers identifying things like ball screen coverages (see the video below) via AI and machine learning.
We’ve seen it in plenty of games and industries other than basketball. Self-learning computers eventually become better at processing information than us. Basketball is no different, and this very idea isn’t exactly unique.
Think about an AI-enabled defensive accounting report. The computer could (theoretically) not just track the end result of a possession, but the entirety of a possession.
If the computer is able to learn what “up-the-line” means when guarding one pass away, we can have exact measurements of one pass away defense for all players.
And if we teach the computer what a ball screen is, we can quantify each player’s ability to fight over those screens.
Machine learning will not only help measure variables more relevant to coaches than proxies (like steals), but also has the advantage of being able to systematically watch thousands of thousands of games and self-learn about the game as it goes.
It’s the eye-test, but with hardware that is much better equipped to perform it.
ICYMI earlier this week
I re-watched the Kansas-Texas Tech game for an article with Jesse Newell
Calvin Wetzel wrote a blog post building off of last week’s newsletter on three-point defense
The top coaches at scoring off of different play types this decade
Kentucky’s hi-lo stack set and cross screen reads